Historically MySQL is great in horizontal READ scale. The scaling in that case is offered by the different number of Replica nodes, no matter if using standard asynchronous replication or synchronous replication.
However those solutions do not offer the same level of scaling for writes operation.
Why? Because the solutions still rely on writing in one single node that works as Primary. Also in case of multi-Primary the writes will be distributed by transaction. In both cases, when using virtually-synchronous replication, the process will require certification from each node and local (by node) write, as such the number of writes are NOT distributed across multiple nodes but duplicated.
The main reason behind this is that MySQL is a relational database system (RDBMS), and any data that is going to be written in it, must respect the RDBMS rules (https://en.wikipedia.org/wiki/Relational_database). In short any data that is written must be consistent with the data present. To achieve that the data needs to be checked with the existing through defined relations and constraints. This action is something that can affect very large datasets and be very expensive. Think about updating a table with millions of rows that refer to another table with another million rows.
An image may help:
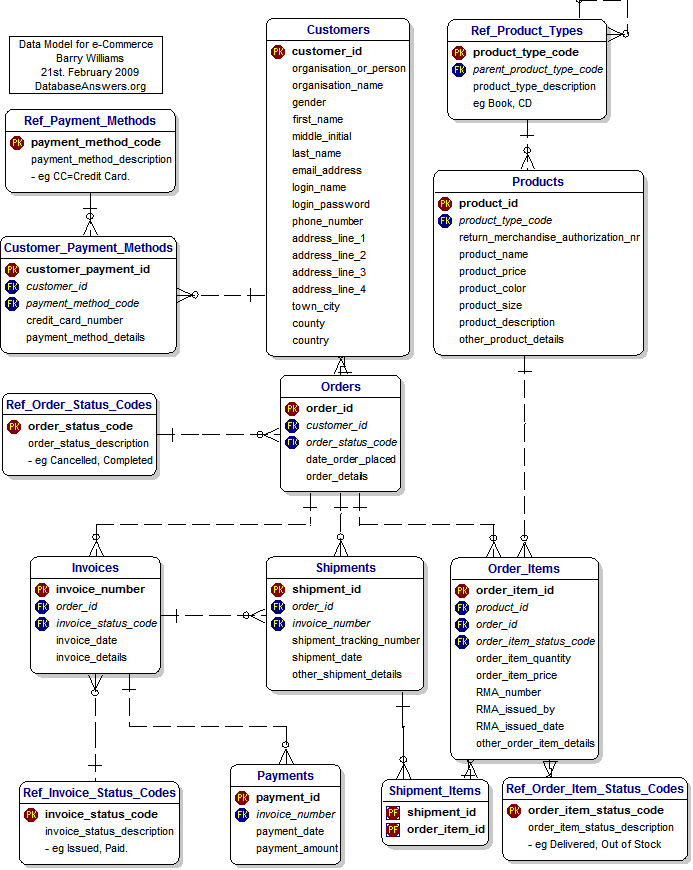
Every time I will insert an order, I must be sure that all the related elements are in place and consistent.
This operation is quite expensive but our database is able to run it in a few milliseconds or less, thanks to several optimizations that allow the node to execute most of them in memory, with none or little access to mass storage.
The key factor is that the whole data structure resides in the same location (node) facilitating the operations.
Once we have understood that, it will also become clear why we cannot have relational data split in multiple nodes and have to distribute writes by table. If I have a node that manages only the items, another the orders, another the payments, I will need to have my solution able to deal with distributed transactions, each of which needs to certify and verify other nodes data.
This level of distribution will seriously affect the efficiency of the operation which will increase the response time significantly. This is it, nothing is impossible however the performances will be so impacted that each operation may take seconds instead milliseconds or fraction of it, unless lifting some of the rules breaking the relational model.
MySQL as well as other RDBMS are designed to work respecting the model and cannot scale in any way by fragmenting and distributing a schema, so what can be done to scale?
The alternative is to split a consistent set of data into fragments. What is a consistent set of data? It all depends on the kind of information we are dealing with. Keeping in mind the example above where we have a shop online serving multiple customers, we need to identify which is the most effective way to split the data.
For instance if we try to split the data by Product Type (Books, CD/DVD, etc) we will have a huge duplication of data related to customers/orders/shipments and so on, all this data is also quite dynamic given I will have customers constantly ordering things.
Why duplicate the data? Because if I do not duplicate that data I will not know if a customer had already bought or not that specific item, or I will have to ask again about the shipment address and so on. Which means also that any time a customer buys something or puts something in the wish list I have to reconcile the data in all my nodes/clusters.
On the other hand if I choose to split my data by country of customer’s residence the only data I will have to duplicate and keep in sync is the one related to the products, of which the most dynamic one will be the number of items in stock. This of course unless I can organize my products by country as well, which is a bit unusual nowadays, but not impossible.
Another possible case is if I am a health organization and I manage several hospitals. As for the example above, it will be easier to split my data by hospital given most of the data related to patients is bound to the hospital itself as well as treatments and any other element related to hospital management. While it will make no sense to split by patient's country of residence.
This technique of splitting the data into smaller pieces is called sharding and at the moment is the only way we have to scale RDBM horizontally.
In the MySQL open source ecosystem we have only two consolidated ways to perform sharding, Vitess and ProxySQL. The first one is a complete solution that takes ownership of your database and manages almost any aspect of its operations in a sharded environment, this includes a lot of specific features for DBA to deal with daily operations like table modifications, backup and more.
While this may look great it also comes with some string attached, including the complexity and proprietary environment. That makes Vitess a good fit for “complex” sharding scenarios where other solutions may not be enough.
ProxySQL does not have a sharding mechanism “per se” but given the way it works and the features it has, allow us to build simple sharding solutions.
It is important to note that most of the DBA operations will still be on DBA to be executed with incremented complexity given the sharding environment.
There is a third option which is application aware sharding.
This solution sees the application be aware of the need to split the data in smaller fragments and internally point the data to different “connectors” who are connected to multiple data sources.
In this case the application is aware of a customer country and will redirect all the operations related to him to the datasource responsible for the specific fragment.
Normally this solution requires a full code re-design and could be quite difficult to achieve when it is injected after the initial code architecture definition.
On the other hand, if done at design it is probably the best solution, because it will allow the application to define the sharding rules and can also optimize the different data sources using different technologies for different uses.
One example could be the use of a RDBMS for most of the Online transaction processing (OLTP) data shared by country, and having the products as distributed memory cache with a different technology. At the same time all the data related to orders, payments and customer history can be consolidated in a data warehouse used to generate reporting.
As said the last one is probably the most powerful, scalable and difficult to design and unfortunately it represents probably less than the 5% of the solution currently deployed.
As well as very few cases are in the need to have a full system/solution to provide scalability with sharding.
By experience, most of the needs for horizontal scaling fell in the simple scenario, where there is the need to achieve sharding and data separation, very often with sharding-nothing architecture. In shared-nothing, each shard can live in a totally separate logical schema instance / physical database server / data center / continent. There is no ongoing need to retain shared access (from between shards) to the other unpartitioned tables in other shards.
The POC
Why this POC?
In the years I have faced a lot of customers that were talking about scaling their database solution and looking at very complex sharding as Vitess as the first and only way to go.
This without even considering if their needs were driving them there for real.
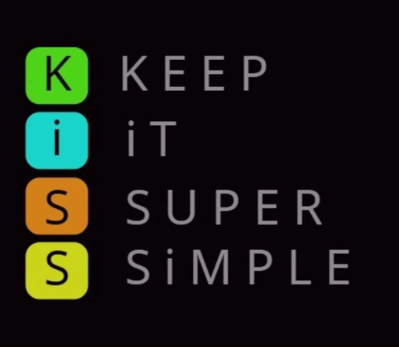
In my experience, and talking with several colleagues, I am not alone, when analyzing the real needs and after discussing with all the parties impacted, only a very small percentage of customers were in the real need of complex solutions. Most of the others were just trying to avoid a project that will implement simple shared-nothing solutions. Why? Because apparently it is simpler to migrate data to a platform that does all for you, than accept a bit of additional work and challenge at the beginning but keep a simple approach. Also going for the last shining things always has its magic.
On top of that, with the rise of Kubernetes and Mysql Operators, a lot of confusion starts to circulate, most of which generate by the total lack of understanding that a Database and a relational database are two separate things. That lack of understanding the difference and the real problems attached to a RDBMS had brought some to talk about horizontal scaling for databases, with a concerning superficiality and without clarifying if they were talking about RDBMS or not. As such some clarification is long due as well as putting back the KISS principle as the main focus.
Given that, I thought that refreshing how ProxySQL could help in building a simple sharding solution may help to clarify the issues, reset the expectations and show how we can do things in a simpler way. (See my old post https://www.percona.com/blog/mysql-sharding-with-proxysql/).
To do so I had built a simple POC that illustrates how you can use Percona Operator for MySQL (POM) and ProxySQL to build a sharded environment with a good level of automation for some standard operations like backup/restore software upgrade and resource scaling.
Why Proxysql?
In the following example we mimic a case where we need a simple sharding solution, which means we just need to redirect the data to different data containers, keeping the database maintenance operations on us. In this common case we do not need to implement a full sharding system such as Vitess.
As illustrated above ProxySQL allows us to set up a common entry point for the application and then redirect the traffic on the base of identified sharding keys. It will also allow us to redirect read/write traffic to the primary and read only traffic to all secondaries.
The other interesting thing is that we can have ProxySQL as part of the application pod, or as an independent service. Best practices indicate that having ProxySQL closer to the application will be more efficient especially if we decide to activate the caching feature.
Why POM
Percona Operator for MySQL comes with three main solutions, Percona Operator for PXC, Percona Operator for MySQL Group Replication and Percona Operator for Percona server. The first two are based on virtually-synchronous replication, and allow the cluster to keep the data state consistent across all pods, which guarantees that the service will always offer consistent data. In K8s context we can see POM as a single service with native horizontal scalability for reads, while for writes we will adopt the mentioned sharding approach.
The other important aspects of using a POM based solution is the automation it comes with. Deploying POM you will be able to set automation for backups, software updates, monitoring (using PMM) and last but not least the possibility to scale UP or DOWN just changing the needed resources.
The elements used
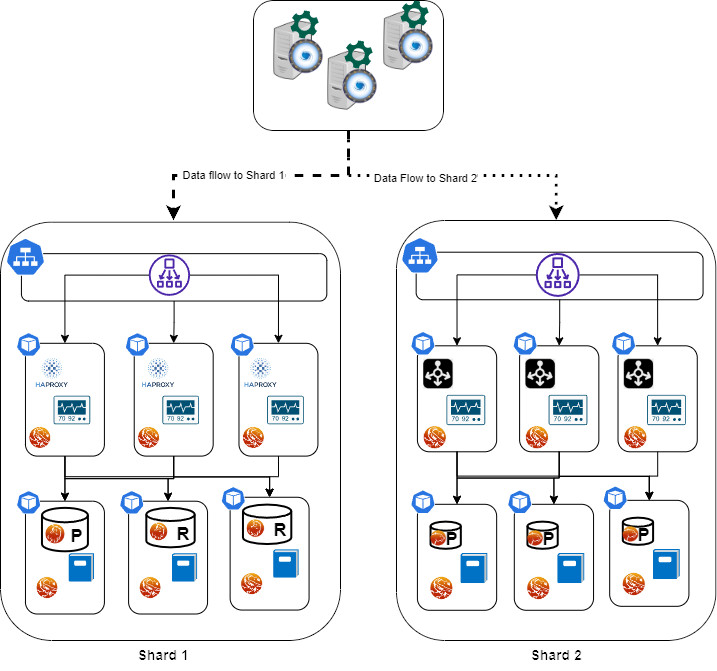
In our POC I will use a modified version of sysbench (https://github.com/Tusamarco/sysbench), that has an additional field continent and I will use that as a sharding key. At the moment and for the purpose of this simple POC I will only have 2 Shards.
As the diagram above illustrates here we have a simple deployment but good enough to illustrate the sharding approach.
We have:
- The application(s) node(s), it is really up to you if you want to test with one application node or more, nothing will change, as well as for the ProxySQL nodes, just keep in mind that if you use more proxysql nodes is better to activate the internal cluster support or use consul to synchronize them.
- Shard 1 is based on POM with PXC, it has:
- Load balancer for service entry point
- Entry point for r/w
- Entry point for read only
- 3 Pods for Haproxy
- Haproxy container
- Pmm agent container
- 3 Pods with data nodes (PXC)
- PXC cluster node container
- Log streaming
- Pmm container
- Backup/restore service
- Shard 2 is based on POM for Percona server and Group Replication (technical preview)
- Load balancer for service entry point
- Entry point for r/w
- Entry point for read only
- 3 Pods for MySQL Router (testing)
- 3 Pods with data nodes (PS with GR)
- PS -GR cluster node container
- Log streaming
- Pmm container
- Backup/restore on scheduler
Now you may have noticed that the representation of the nodes are different in size, this is not a mistake while drawing. It indicates that I have allocated more resources (CPU and Memory) to shard1 than shard2. Why? Because I can and I am simulating a situation where a shard2 gets less traffic, at least temporarily, as such I do not want to give it the same resources as shard1. I will eventually increase them if I see the needs.
The settings
Data layer
Let us start with the easy one, the Data layer configuration. Configuring correctly the environment is the key, and to do so I am using a tool that I wrote specifically to calculate the needed configuration in K8s POM environment, you can find it here (https://github.com/Tusamarco/mysqloperatorcalculator).
Once you have compiled it and run you can simply ask what “dimensions” are supported, or you can define a custom level of resources, but you will still need to indicate the level of expected load. In any case please refer to the README in the repository which has all the instructions.
The full cr.yaml for PXC shard1 is here, while the one for PS-GR here.
For Shard 1: I asked for resources to cover traffic of type 2 (Light OLTP), configuration type 5 (2XLarge) 1000 connections.
For Shard2: I ask for resources to cover traffic of type 2 (Light OLTP), configuration type 2 (Small), 100 connections.
Once you have the CRs defined you can follow the official guidelines to set the environment up ( PXC (https://docs.percona.com/percona-operator-for-mysql/pxc/index.html), PS (https://docs.percona.com/percona-operator-for-mysql/ps/index.html)
It is time now to see the Proxysql settings.
ProxySQL and Sharding rules
As mentioned before we are going to test the load sharding by continent, and as also mentioned before we know that ProxySQL will not provide additional help to automatically manage the sharded environment.
Given that one way to do it is to create a DBA account per shard, or to inject shard information in the commands while executing.
I will use the less comfortable one just to prove if it works, the different DBA accounts.
We will have 2 shards, the sharding key is the continent field, and the continents will be grouped as follows:
- Shard 1:
- Asia
- Africa
- Antarctica
- Europe
- North America
- Shard 2:
The DBAs users:
The application user:
The host groups will be:
- Shard 1
- 100 Read and Write
- 101 Read only
- Shard 2
- 200 Read and Write
- 201 Read only
Once that is defined, we need to identify which query rules will serve us and how.
What we want is to redirect all the incoming queries for:
- Asia, Africa, Antarctica, Europe and North America to shard 1.
- Oceania and South America to shard 2
- Split the queries in R/W and Read only
- Prevent the execution of any query that do not have a shard key
- Backup data at regular interval and store it in a safe place
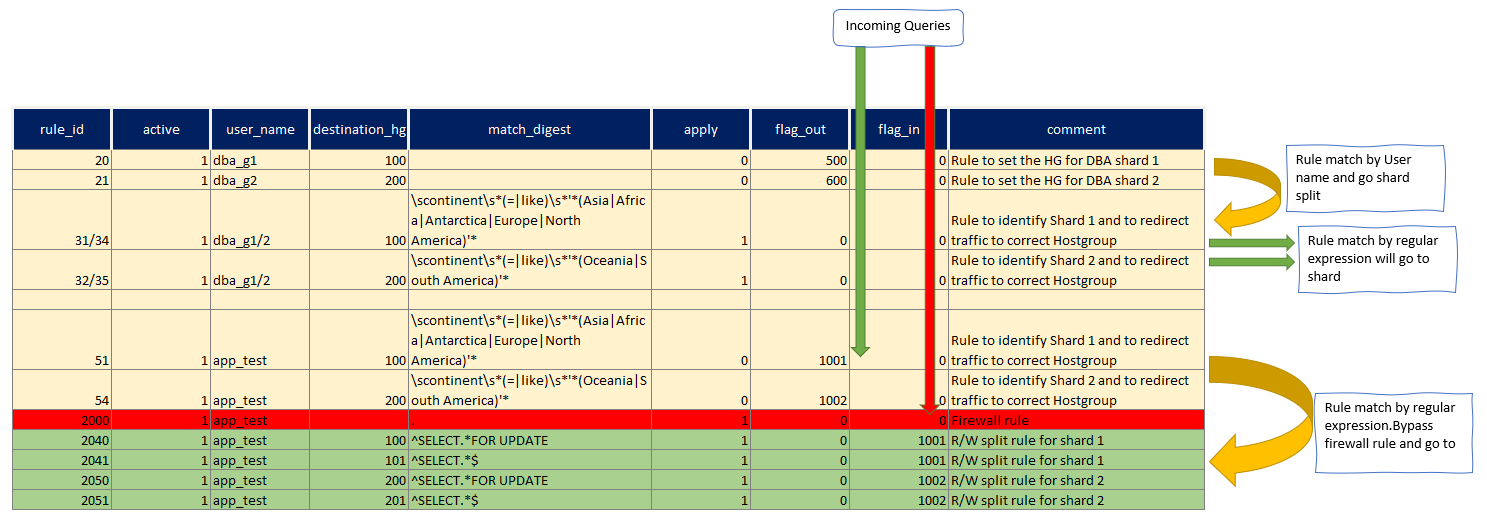
Given the above we first define the rules for the DBAs accounts:
We set the Hostgroup for each DBA and then if the query matches the sharding rule we redirect it to the proper sharding, otherwise the HG will remain as set.
This allows us to execute queries like CREATE/DROP table on our shard without problem, but will allow us to send data where needed.
For instance the one below is the output of the queries that sysbench will run.
Prepare:
INSERT INTO windmills_test1 /* continent=Asia */ (uuid,millid,kwatts_s,date,location,continent,active,strrecordtype) VALUES(UUID(), 79, 3949999,NOW(),'mr18n2L9K88eMlGn7CcctT9RwKSB1FebW397','Asia',0,'quq')
In this case I have the application simply injecting a comment in the INSERT SQL declaring the shard key, given I am using the account dba_g1 to create/prepare the schemas, rules 32/32 will be used and give I have sett apply=1, ProxySQL will exit the query rules parsing and send the command to relevant hostgroup.
Run:
SELECT id, millid, date,continent,active,kwatts_s FROM windmills_test1 WHERE id BETWEEN ? AND ? AND continent='South America'
SELECT SUM(kwatts_s) FROM windmills_test1 WHERE id BETWEEN ? AND ? and active=1 AND continent='Asia'
SELECT id, millid, date,continent,active,kwatts_s FROM windmills_test1 WHERE id BETWEEN ? AND ? AND continent='Oceania' ORDER BY millid
SELECT DISTINCT millid,continent,active,kwatts_s FROM windmills_test1 WHERE id BETWEEN ? AND ? AND active =1 AND continent='Oceania' ORDER BY millid
UPDATE windmills_test1 SET active=? WHERE id=? AND continent='Asia'
UPDATE windmills_test1 SET strrecordtype=? WHERE id=? AND continent='North America'
DELETE FROM windmills_test1 WHERE id=? AND continent='Antarctica'
INSERT INTO windmills_test1 /* continent=Antarctica */ (id,uuid,millid,kwatts_s,date,location,continent,active,strrecordtype) VALUES (?, UUID(), ?, ?, NOW(), ?, ?, ?,?) ON DUPLICATE KEY UPDATE kwatts_s=kwatts_s+1
The above are executed during the tests.
In all of them the sharding key is present, either in the WHERE clause OR as comment.
Of course if I execute one of them without the sharding key, the firewall rule will stop the query execution, ie:
mysql> SELECT id, millid, date,continent,active,kwatts_s FROM windmills_test1 WHERE id BETWEEN ? AND ?;
ERROR 1148 (42000): It is impossible to redirect this command to a defined shard. Please be sure you Have the Continent definition in your query, or that you use a defined DBA account (dba_g{1/2})
Check here for full command list
Setting up the dataset
Once we have the rules set it is time to setup the schemas and the data using sysbench (https://github.com/Tusamarco/sysbench), remember to use windmills_sharding tests.
The first operation is to build the schema on SHARD2 without filling it with data. This is a DBA action as such we will execute it using the dba_g2 account:
sysbench ./src/lua/windmills_sharding/oltp_read.lua --mysql-host=10.0.1.96 --mysql-port=6033 --mysql-user=dba_g2 --mysql-password=xxx --mysql-db=windmills_large --mysql_storage_engine=innodb --db-driver=mysql --tables=4 --table_size=0 --table_name=windmills --mysql-ignore-errors=all --threads=1 prepare
Setting table_size and pointing to the ProxySQL IP/port will do, and I will have:
mysql> select current_user(), @@hostname;
+----------------+-------------------+
| current_user() | @@hostname |
+----------------+-------------------+
| dba_g2@% | ps-mysql1-mysql-0 |
+----------------+-------------------+
1 row in set (0.01 sec)
mysql> use windmills_large;
Database changed
mysql> show tables;
+---------------------------+
| Tables_in_windmills_large |
+---------------------------+
| windmills1 |
| windmills2 |
| windmills3 |
| windmills4 |
+---------------------------+
4 rows in set (0.01 sec)
mysql> select count(*) from windmills1;
+----------+
| count(*) |
+----------+
| 0 |
+----------+
1 row in set (0.09 sec)
All set but empty.
Now let us do the same but with the other DBA user:
sysbench ./src/lua/windmills_sharding/oltp_read.lua --mysql-host=10.0.1.96 --mysql-port=6033 --mysql-user=dba_g1 --mysql-password=xxx --mysql-db=windmills_large --mysql_storage_engine=innodb --db-driver=mysql --tables=4 --table_size=400 --table_name=windmills --mysql-ignore-errors=all --threads=1 prepare
If I do now the select above with user dba_g2:
mysql> select current_user(), @@hostname;select count(*) from windmills1;
+----------------+-------------------+
| current_user() | @@hostname |
+----------------+-------------------+
| dba_g2@% | ps-mysql1-mysql-0 |
+----------------+-------------------+
1 row in set (0.00 sec)
+----------+
| count(*) |
+----------+
| 113 |
+----------+
1 row in set (0.00 sec)
While If I reconnect and use dba_g1:
mysql> select current_user(), @@hostname;select count(*) from windmills1;
+----------------+--------------------+
| current_user() | @@hostname |
+----------------+--------------------+
| dba_g1@% | mt-cluster-1-pxc-0 |
+----------------+--------------------+
1 row in set (0.00 sec)
+----------+
| count(*) |
+----------+
| 287 |
+----------+
1 row in set (0.01 sec)
I can also check on ProxySQL to see which rules were utilized:
select active,hits,destination_hostgroup, mysql_query_rules.rule_id, match_digest, match_pattern, replace_pattern, cache_ttl, apply,flagIn,flagOUT FROM mysql_query_rules NATURAL JOIN stats.stats_mysql_query_rules ORDER BY mysql_query_rules.rule_id;
+------+-----------------------+---------+---------------------+----------------------------------------------------------------------------+-------+--------+---------+
| hits | destination_hostgroup | rule_id | match_digest | match_pattern | apply | flagIN | flagOUT |
+------+-----------------------+---------+---------------------+----------------------------------------------------------------------------+-------+--------+---------+
| 3261 | 100 | 20 | NULL | NULL | 0 | 0 | 500 |
| 51 | 200 | 21 | NULL | NULL | 0 | 0 | 600 |
| 2320 | 100 | 31 | NULL | scontinents*(=|like)s*'*(Asia|Africa|Antarctica|Europe|North America)'* | 1 | 500 | 0 |
| 880 | 200 | 32 | NULL | scontinents*(=|like)s*'*(Oceania|South America)'* | 1 | 500 | 0 |
| 0 | 100 | 34 | NULL | scontinents*(=|like)s*'*(Asia|Africa|Antarctica|Europe|North America)'* | 1 | 600 | 0 |
| 0 | 200 | 35 | NULL | scontinents*(=|like)s*'*(Oceania|South America)'* | 1 | 600 | 0 |
| 2 | 100 | 51 | NULL | scontinents*(=|like)s*'*(Asia|Africa|Antarctica|Europe|North America)'* | 0 | 0 | 1001 |
| 0 | 200 | 54 | NULL | scontinents*(=|like)s*'*(Oceania|South America)'* | 0 | 0 | 1002 |
| 0 | 100 | 60 | NULL | NULL | 0 | 50 | 1001 |
| 0 | 200 | 62 | NULL | NULL | 0 | 60 | 1002 |
| 7 | NULL | 2000 | . | NULL | 1 | 0 | NULL |
| 0 | 100 | 2040 | ^SELECT.*FOR UPDATE | NULL | 1 | 1001 | NULL |
| 2 | 101 | 2041 | ^SELECT.*$ | NULL | 1 | 1001 | NULL |
| 0 | 200 | 2050 | ^SELECT.*FOR UPDATE | NULL | 1 | 1002 | NULL |
| 0 | 201 | 2051 | ^SELECT.*$ | NULL | 1 | 1002 | NULL |
+------+-----------------------+---------+---------------------+----------------------------------------------------------------------------+-------+--------+---------+
Running the application
Now that the data load test was successful, let us do the real load following the indication as above but use 80 Tables and just a bit more records like 20000, nothing huge.
Once the data is loaded we will have the 2 shards with different numbers of records, if all went well the shard2 should have ¼ of the total and shard1 ¾ .
When load is over I have as expected:
mysql> select current_user(), @@hostname;select count(*) as shard1 from windmills_large.windmills80;select /* continent=shard2 */ count(*) as shard2 from windmills_large.windmills80;
+----------------+--------------------+
| current_user() | @@hostname |
+----------------+--------------------+
| dba_g1@% | mt-cluster-1-pxc-0 |
+----------------+--------------------+
1 row in set (0.00 sec)
+--------+
| shard1 |
+--------+
| 14272 | ← Table windmills80 in SHARD1
+--------+
+--------+
| shard2 |
+--------+
| 5728 | ← Table windmills80 in SHARD2
+--------+
As you may have already noticed, I used a trick to query the other shard using the dba_g1 user, I just passed in the query the shard2 definition as a comment. That is all we need.
Let us execute the run command for writes in sysbench and see what happens.
The first thing we can notice while doing writes is the query distribution:
+--------+-----------+----------------------------------------------------------------------------+----------+--------+----------+----------+--------+---------+-------------+---------+
| weight | hostgroup | srv_host | srv_port | status | ConnUsed | ConnFree | ConnOK | ConnERR | MaxConnUsed | Queries |
+--------+-----------+----------------------------------------------------------------------------+----------+--------+----------+----------+--------+---------+-------------+---------+
| 10000 | 100 | ac966f7d46c04400fb92a3603f0e2634-193113472.eu-central-1.elb.amazonaws.com | 3306 | ONLINE | 24 | 0 | 138 | 66 | 25 | 1309353 |
| 100 | 101 | a5c8836b7c05b41928ca84f2beb48aee-1936458168.eu-central-1.elb.amazonaws.com | 3306 | ONLINE | 0 | 0 | 0 | 0 | 0 | 0 |
| 10000 | 200 | a039ab70e9f564f5e879d5e1374d9ffa-1769267689.eu-central-1.elb.amazonaws.com | 3306 | ONLINE | 24 | 1 | 129 | 66 | 25 | 516407 |
| 10000 | 201 | a039ab70e9f564f5e879d5e1374d9ffa-1769267689.eu-central-1.elb.amazonaws.com | 6447 | ONLINE | 0 | 0 | 0 | 0 | 0 | 0 |
+--------+-----------+----------------------------------------------------------------------------+----------+--------+----------+----------+--------+---------+-------------+---------+
Where we can notice that the load in connection is evenly distributed, while the load is mainly going to shard1 as we expected given we have an unbalanced sharding by design.
At MySQL level we had:
Questions

Com Type

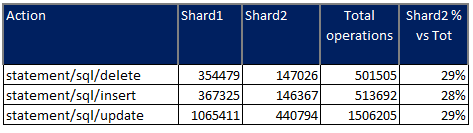
The final point is, what is the gain using this sharding approach?
Well we still need to consider the fact we are testing on a very small set of data, however if we can already identify some benefit here, that will be an interesting result.
Let see the write operations with 24 and 64 threads:
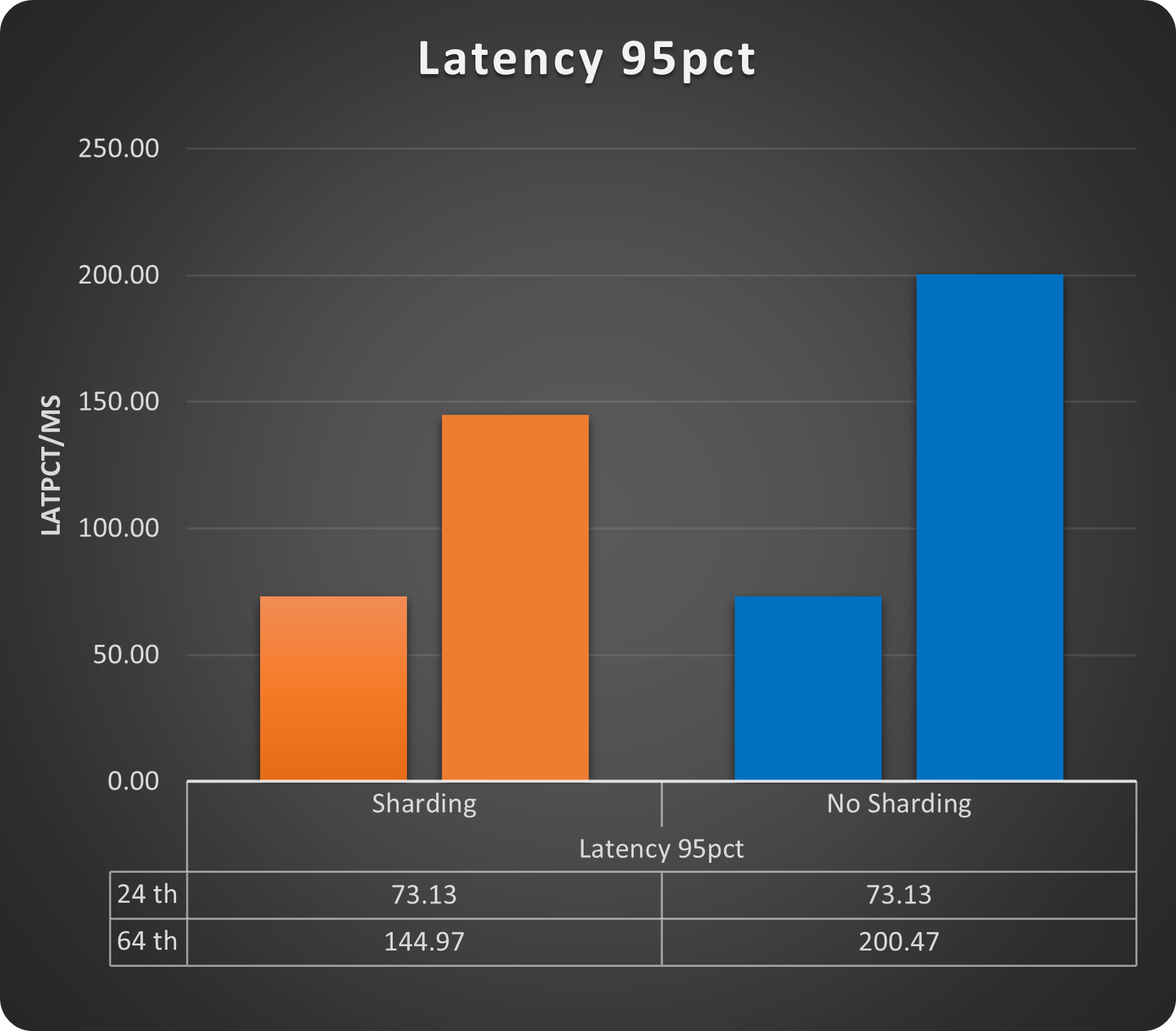
We get a gain of ~33% just using sharding, while for latency we do not have a cost on the contrary also with small load increase we can see how the sharded solution performs better. Of course we are still talking about low number of rows and running threads but gain is there.
Backup
The backup and restore operation when using POM is completely managed by the operator (see instructions in the POM documentation https://docs.percona.com/percona-operator-for-mysql/pxc/backups.html and https://docs.percona.com/percona-operator-for-mysql/ps/backups.html ).
The interesting part is that we can have multiple kind of backup solution, like:
- On demand
- Scheduled
- Full Point in time recovery with log streaming
Automation will allow us to set schedule as simple as this:
schedule:
- name: "sat-night-backup"
schedule: "0 0 * * 6"
keep: 3
storageName: s3-eu-west
- name: "daily-backup"
schedule: "0 3 * * *"
keep: 7
storageName: s3-eu-west
Or if you want to run the on demand:
kubectl apply -f backup.yaml
Where the backup.yaml file has very simple informations:
apiVersion: ps.percona.com/v1alpha1
kind: PerconaServerMySQLBackup
metadata:
name: ps-gr-sharding-test-2nd-of-may
# finalizers:
# - delete-backup
spec:
clusterName: ps-mysql1
storageName: s3-ondemand
Using both methods we will be able to soon have a good set of backups like:
POM (PXC)
cron-mt-cluster-1-s3-eu-west-20234293010-3vsve mt-cluster-1 s3-eu-west s3://mt-bucket-backup-tl/scheduled/mt-cluster-1-2023-04-29-03:00:10-full Succeeded 3d9h 3d9h
cron-mt-cluster-1-s3-eu-west-20234303010-3vsve mt-cluster-1 s3-eu-west s3://mt-bucket-backup-tl/scheduled/mt-cluster-1-2023-04-30-03:00:10-full Succeeded 2d9h 2d9h
cron-mt-cluster-1-s3-eu-west-2023513010-3vsve mt-cluster-1 s3-eu-west s3://mt-bucket-backup-tl/scheduled/mt-cluster-1-2023-05-01-03:00:10-full Succeeded 33h 33h
cron-mt-cluster-1-s3-eu-west-2023523010-3vsve mt-cluster-1 s3-eu-west s3://mt-bucket-backup-tl/scheduled/mt-cluster-1-2023-05-02-03:00:10-full Succeeded 9h 9h
POM (PS) *
NAME STORAGE DESTINATION STATE COMPLETED AGE
ps-gr-sharding-test s3-ondemand s3://mt-bucket-backup-tl/ondemand/ondemand/ps-mysql1-2023-05-01-15:10:04-full Succeeded 21h 21h
ps-gr-sharding-test-2nd-of-may s3-ondemand s3://mt-bucket-backup-tl/ondemand/ondemand/ps-mysql1-2023-05-02-12:22:24-full Succeeded 27m 27m
To note that as DBA, we still need to validate the backups with a restore procedure, that part is not automated (yet).
*Note that Backup for POM PS is available only on demand given the solution is still in technical preview
When will this solution fit in?
As mentioned multiple times, this solution can cover simple cases of sharding, better if you have shared-nothing.
It also requires work from the DBA side in case of DDL operations or resharding.
You also need to be able to change some SQL code in order to be sure to have present the sharding key/information, in any SQL executed.
When will this solution not fit in?
There are several things that could prevent you to use this solution, the most common ones are:
- You need to query multiple shards at the same time. This is not possible with ProxySQL
- You do not have a DBA to perform administrative work and need to rely on automated system
- Distributed transaction cross shard
- No access to SQL code.
Conclusions
We do not have the Amletic dilemma about sharding or not sharding.
When using a RDBMS like MySQL, if you need horizontal scalability, you need to shard.
The point is there is no magic wand or solution, moving to sharding is an expensive and impacting operation. If you choose it at the beginning, before doing any application development, the effort can be significantly less.
Doing sooner will also allow you to test proper solutions, where proper is a KISS solution, always go for the less complex things, in 2 years you will be super happy about your decision.
If instead you must convert a current solution, then prepare for a bloodshed, or at least for a long journey.
In any case we need to keep in mind few key points:
- Do not believe most of the articles on the internet that promise you infinite scalability for your database. If there is no distinction in the article about a simple database and a RDBMS, run away.
- Do not go for the last shiny things just because they shine. Test them and evaluate IF it makes sense for you. Better to spend a quarter testing now a few solutions, than fight for years with something that you do not fully comprehend.
- Using containers/operators/kubernetes do not scale per se, you must find a mechanism to have the solution scaling, there is absolutely NO difference with premises. What you may get is a good level of automation, however that will come with a good level of complexity, it is up to you to evaluate if it makes sense or not.
As said at the beginning for MYSQL the choice is limited. Vitess is the full complete solution, with a lot of coding to provide you a complete platform to deal with your scaling needs.
However do not be so fast to exclude ProxySQL as possible solutions. There are out there already many using it also for sharding.
This small POC used a synthetic case, but it also shows that with just 4 rules you can achieve a decent solution. A real scenario could be a bit more complex … or not.
References
Vitess (https://vitess.io/docs/)
ProxySQL (https://proxysql.com/documentation/)
Firewalling with ProxySQL (https://www.tusacentral.com/joomla/index.php/mysql-blogs/197-proxysql-firewalling)
Sharding: